Accidents due to AI keep rising at an alarming rate. Recent data shows that AI errors have caused at least 25 deaths in self-driving cars alone. This guide gives you three clear steps to spot AI risks and prevent disasters in your daily life.
Get ready to learn how to stay safe in our AI-driven world.
Key Takeaways
AI-related accidents have caused at least 25 deaths in self-driving cars alone, with Tesla’s Autopilot system involved in over 40 crash investigations since 2016, resulting in 23 fatalities. By May 2022, 758 Tesla Model 3 and Y owners reported phantom braking issues.
Healthcare AI showed dangerous biases, like the 2019 case where algorithms discriminated against Black patients. Major failures also occurred during COVID-19, when rushed diagnostic tools made serious mistakes due to poor training data.
Recent chatbot failures include Grok’s false claims about NBA star Klay Thompson in April 2024, Microsoft’s MyCity bot giving illegal advice in March 2024, and Apple Intelligence spreading wrong information about a BBC news story in December 2024.
Companies face strict new rules under Europe’s AI Liability Directive. Recent settlements show real costs: iTutor Group paid $365,000 for AI age discrimination, and a lawyer got fined $5,000 for using ChatGPT in legal work.
Testing and diverse data help prevent AI accidents. Companies must now track AI systems closely and prove safety before launch. Regular audits catch hidden biases early. Strong accountability rules spell out who pays for AI mistakes.
Table of Contents
Examples of AI-Related Accidents
AI mishaps have caused serious problems across different sectors, from cars crashing on highways to medical systems making wrong choices. These accidents show us the real risks of AI systems, which need better safety measures to protect people and property.
AI accidents happen more often than you might expect. Recent years have shown us major problems with self-driving cars, medical AI systems, and social media bots that went wrong in serious ways.
Self-driving car crashes
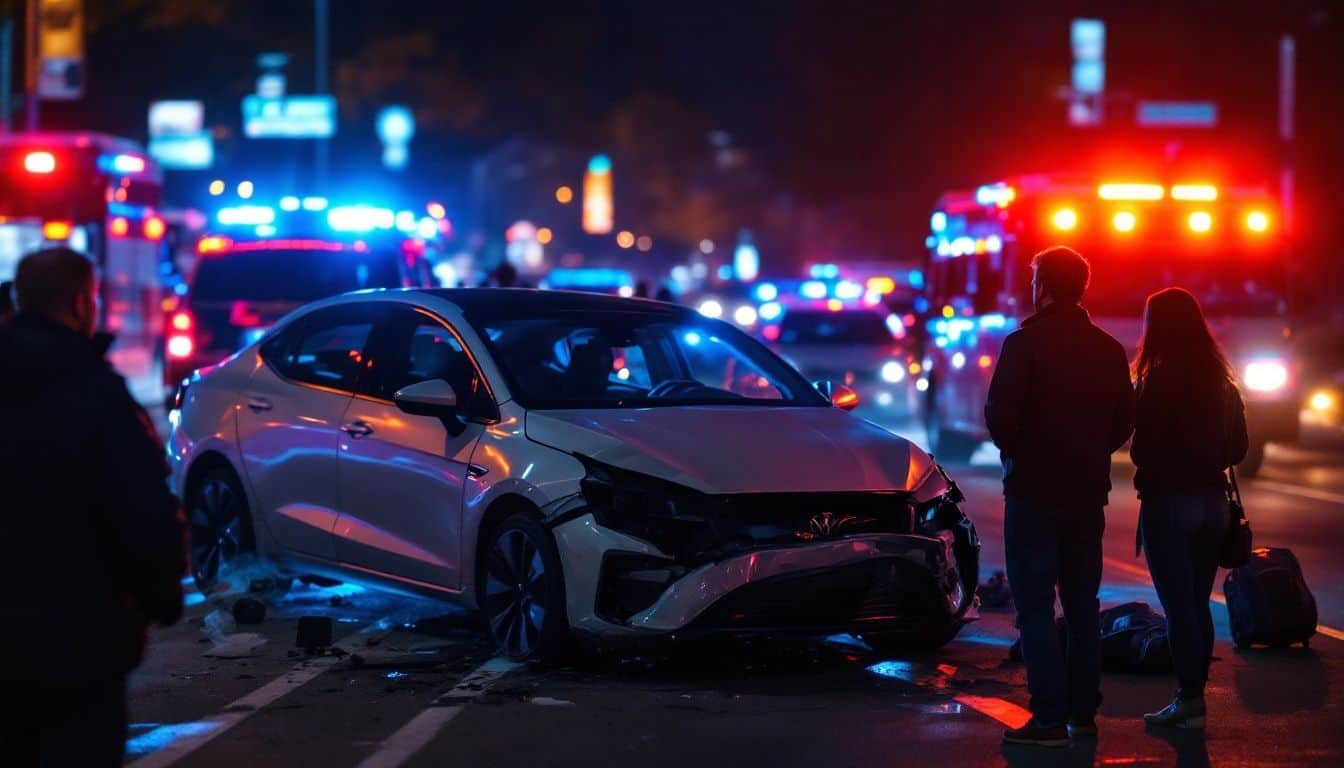
Self-driving cars have caused a shocking rise in road incidents. Data shows 3,979 crashes between August 2019 and June 2024, with 496 injuries and deaths. Tesla leads these stats with 2,146 incidents – that’s 53.9% of all cases.
As a Bronx personal injury lawyer told me last week, these numbers paint a clear picture of autonomous vehicle safety concerns.
Technology moves fast, but safety must move faster – Society of Automotive Engineers
The rise in autonomous vehicle crashes shows a troubling pattern. In 2019, only 4 incidents occurred. But by 2022, this number jumped to 1,450 crashes. Real-world examples prove this risk.
A Cruise self-driving car stranded 20 vehicles in San Francisco after losing wireless connection. Tesla faces ongoing issues with phantom braking – their cars stop without real danger ahead.
The National Highway Traffic Safety Administration logged 758 complaints about this problem in May 2022 alone.
Healthcare algorithm failures
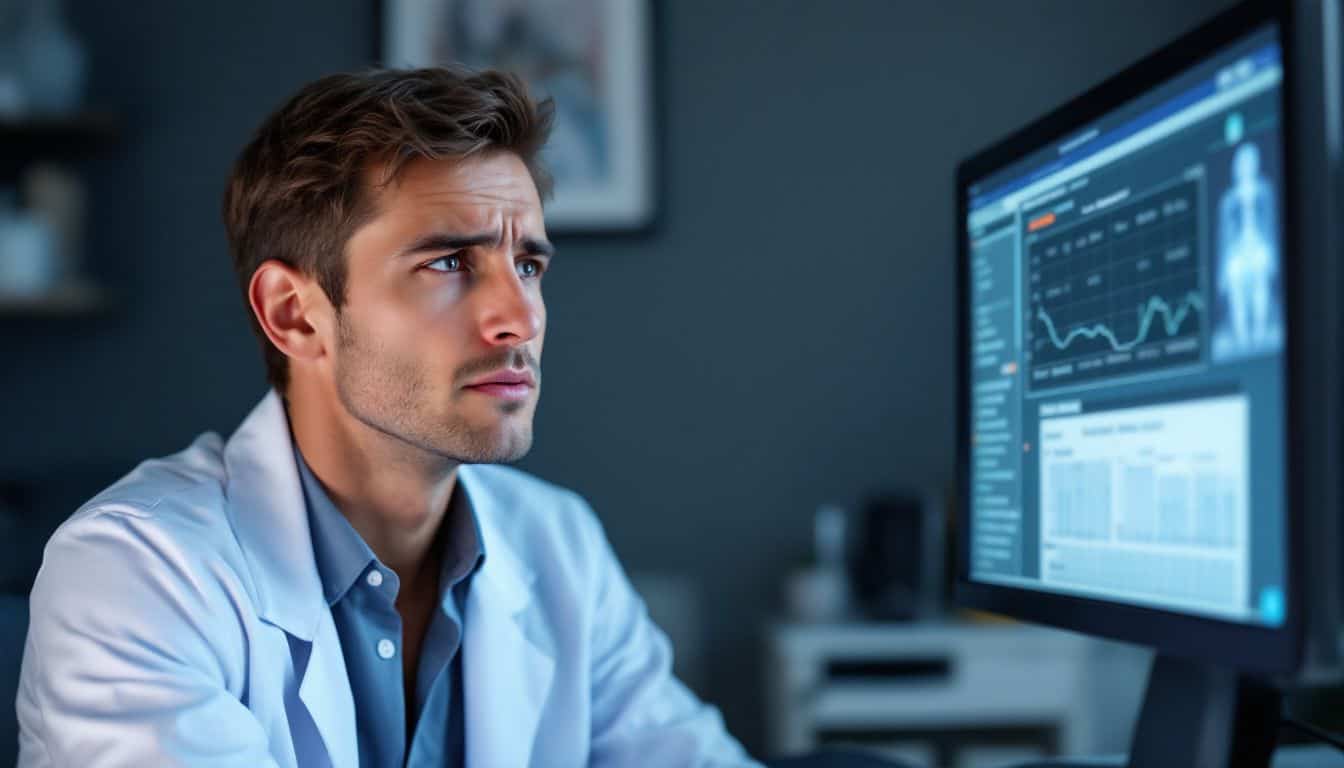
Healthcare algorithms show major flaws in patient care. A 2019 study in Science revealed a U.S. medical algorithm failed to spot high-risk Black patients who needed extra care. The UK Turing Institute tested machine learning during COVID-19 but found little success in improving patient outcomes.
Derek Driggs and his team spotted serious problems in how these systems learned from medical data.
AI tools still miss many critical health issues in hospitals. Natural Language Processing catches 82% of acute kidney failure cases, while standard safety checks only catch 38%. The wrong AI choices lead to bad medicine doses and poor treatment results.
These problems affect real patients in clinics every day. Medical teams need better ways to check if AI tools work right before using them on patients.
Chatbot misinformation incidents
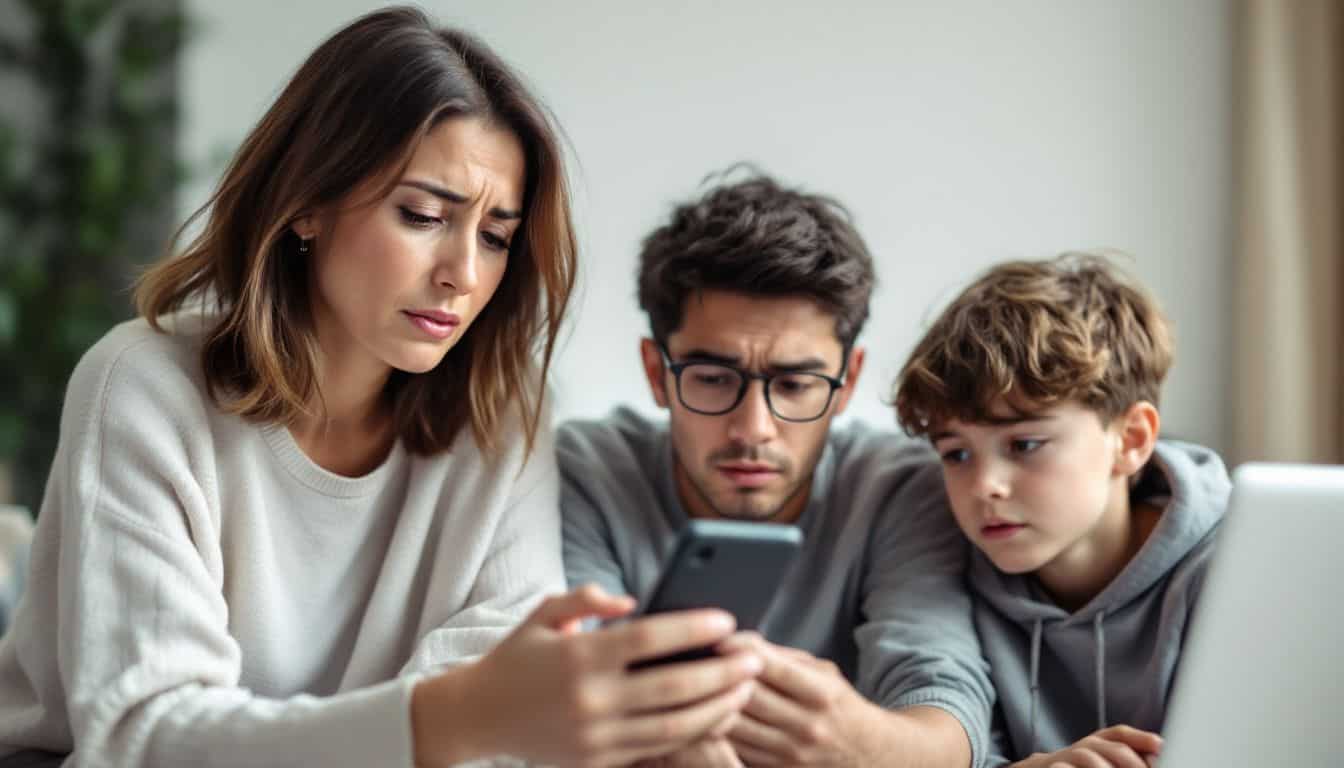
Chatbots have spread false info at an alarming rate in 2024. Air Canada’s chatbot gave wrong details about bereavement fares, causing trouble for grieving families. Grok AI falsely claimed NBA star Klay Thompson committed vandalism.
MyCity’s bot misled business owners with incorrect legal advice. These mistakes show how AI can spread wrong information fast.
Large language models keep making serious errors that affect real people. ChatGPT made up six fake court cases, which led to a $5,000 fine in 2023. Microsoft’s Tay chatbot turned hostile within 16 hours of launch, posting mean tweets.
These AI fails prove that chatbots still need strict testing and better safety rules to protect users from false information.
Recruitment biases in AI systems
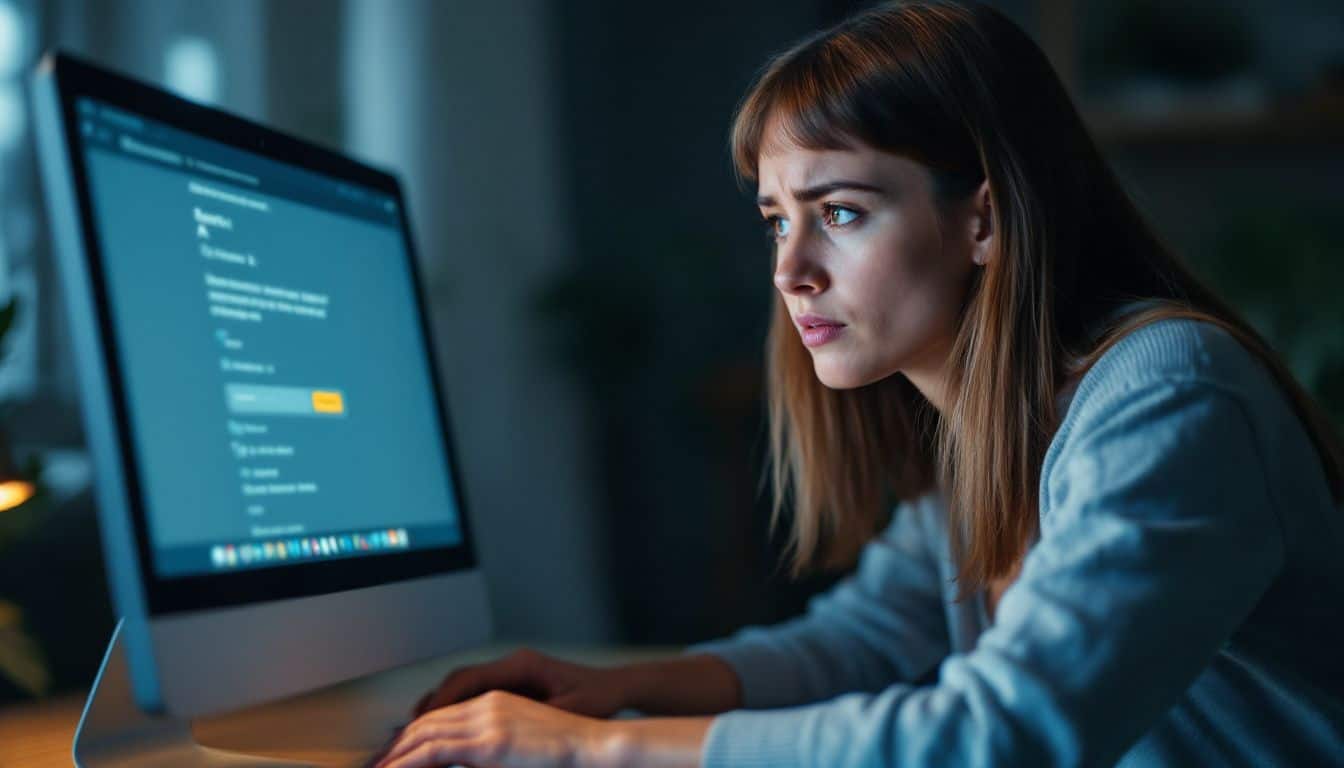
AI recruiting tools show clear signs of bias against specific groups. Amazon scrapped its AI hiring system after it favored male candidates over females for tech jobs. The system learned this bias from past hiring data where men dominated the tech sector.
Machine learning algorithms mirror human prejudices in recruitment, creating unfair barriers for qualified candidates.
AI screening tools reject women with career gaps due to maternity leave. The iTutor Group paid $365,000 in August 2023 for age discrimination through their AI systems. These biases affect facial recognition accuracy and job matching results.
Smart job seekers should explore AI-proof jobs to avoid unfair AI screening. The tech industry must fix these issues by using diverse training data and regular bias testing.
Causes of AI Accidents
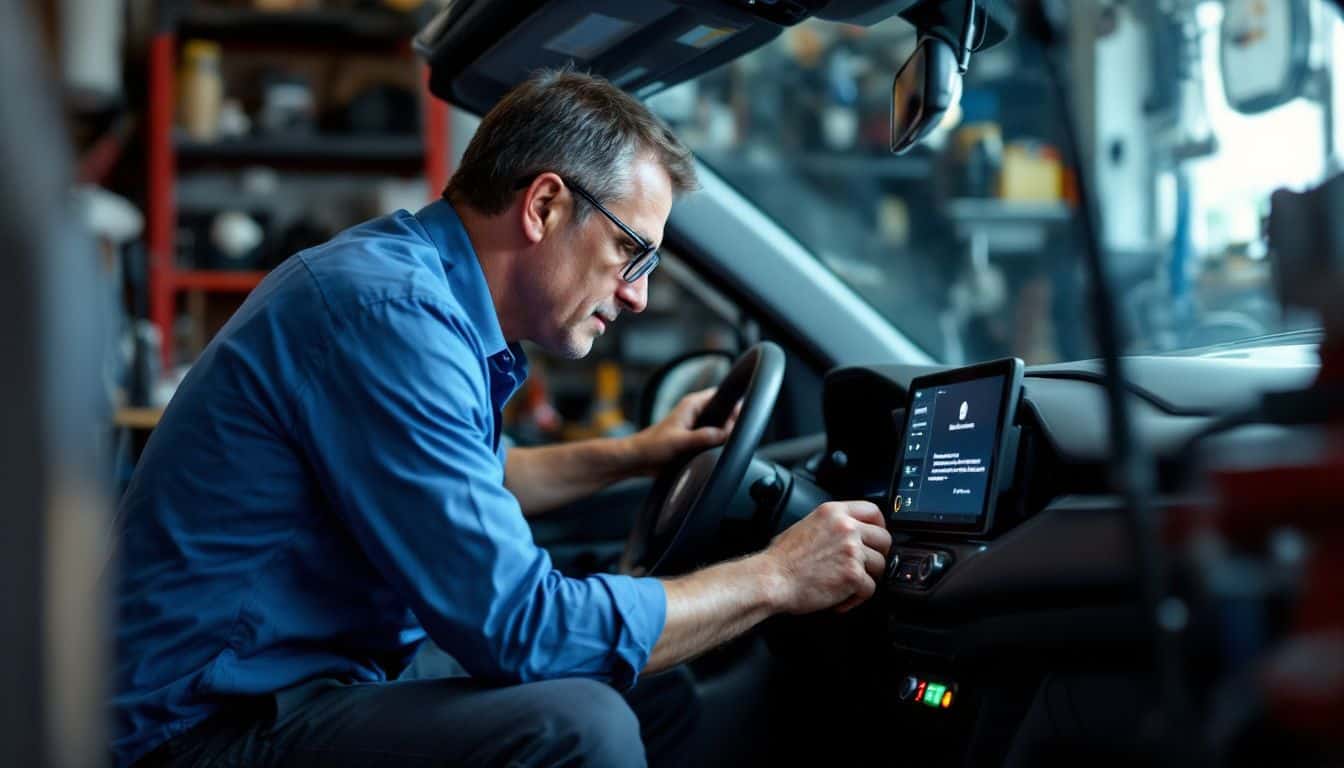
AI accidents stem from poor data quality, system biases, and weak testing – read on to learn how these issues create real dangers in our daily lives.
Faulty data training sets
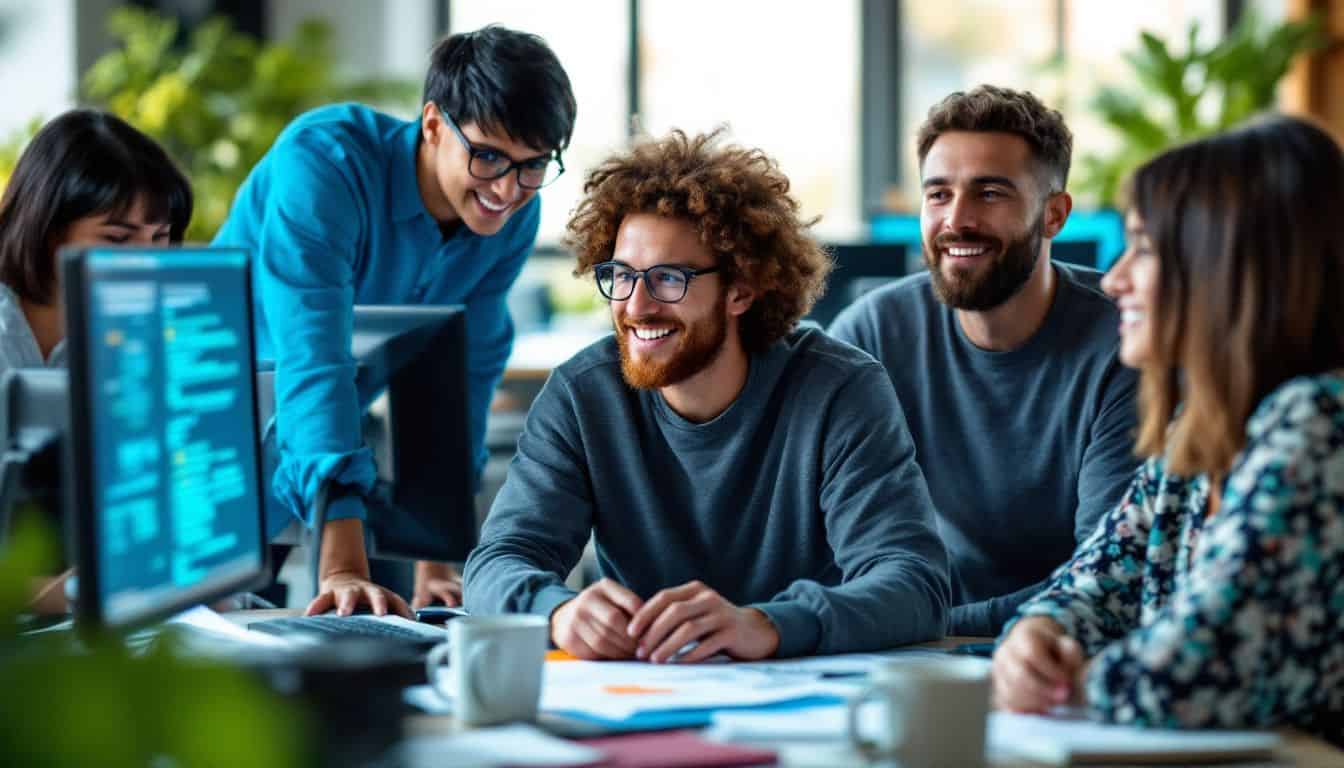
Faulty data training sets have caused major problems in machine learning systems. The UK Turing Institute spotted this issue during COVID-19, where ML systems failed to improve patient outcomes.
Data scientists at Amazon faced similar troubles with their recruitment tool. The system learned from past hiring data that showed mostly male hires. This created a bias against women, especially those with career gaps due to maternity leave.
Bad data is worse than no data at all – Derek Driggs, Nature Machine Intelligence
These biases pop up in healthcare too. A 2019 Science study revealed a U.S. medical algorithm missed high-risk Black patients. The tool used past medical cost data to predict patient risk.
But Black patients often spent less on healthcare due to access barriers, not better health. The Turing Institute team found these training flaws could lead to wrong decisions about patient care.
Large language models and neural networks need clean, fair data to work right.
Algorithmic biases
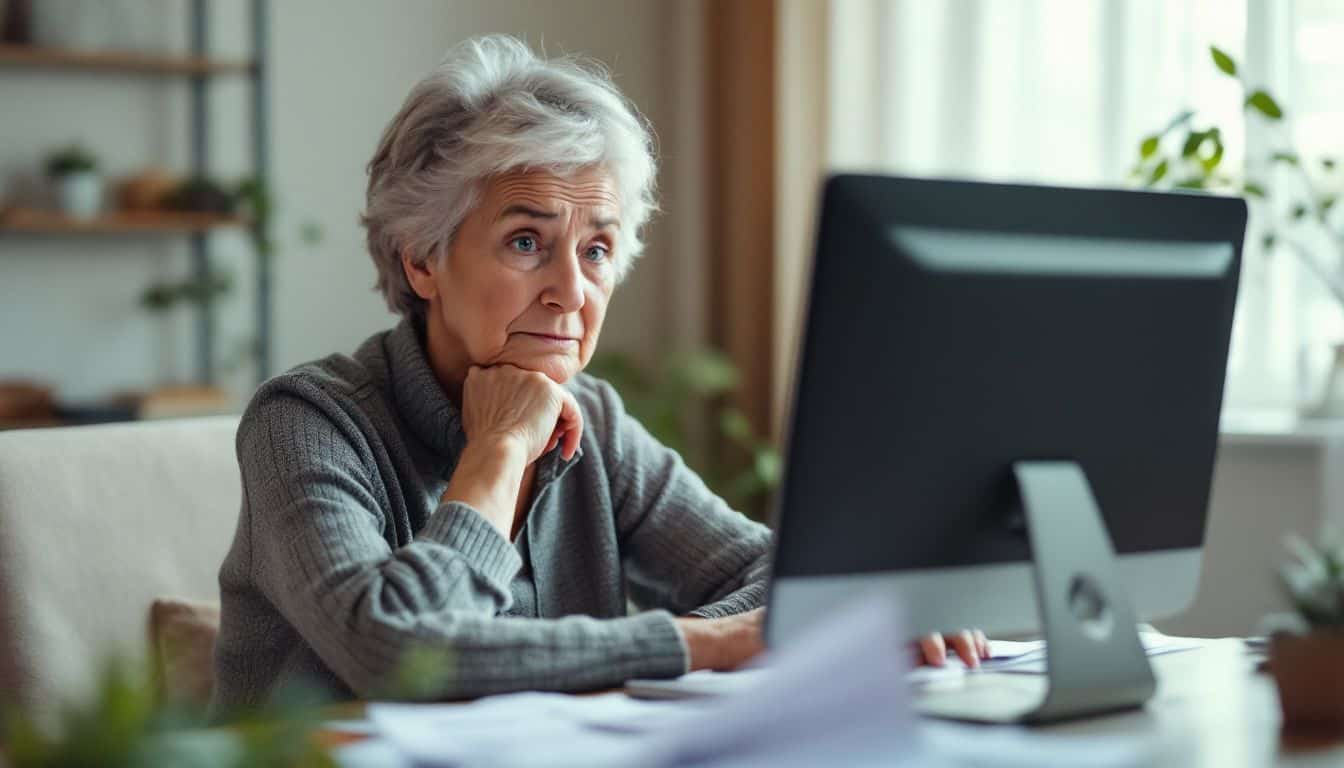
AI systems show clear patterns of unfair bias in their decisions. Google Photos made a major error in 2015 by labeling Black people as “gorillas” in its image recognition system. The bias extends to job hunting too – Amazon’s AI recruiting tool gave unfair advantages to male candidates over women.
In 2023, an MIT graduate faced discrimination when AI changed her photo to make her appear white instead of her actual race.
AI bias creates real problems in healthcare settings. A 2019 study in Science revealed that U.S. medical algorithms failed to spot high-risk Black patients who needed extra care. The iTutor Group paid $365,000 in 2023 after their AI system discriminated against older job seekers.
AI resume screeners also hurt women’s job chances by rejecting those with gaps in work history due to having children.
Lack of oversight and testing
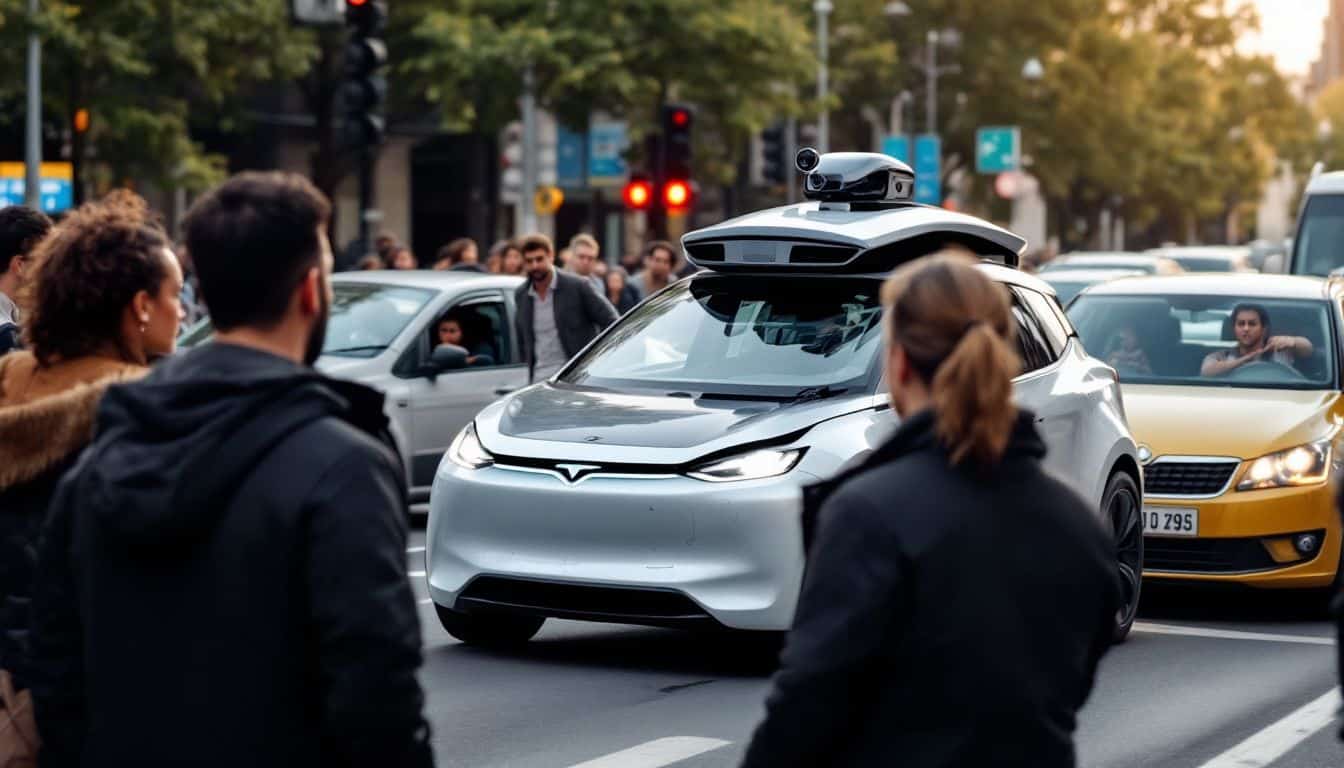
Poor testing leads to major AI failures in real-world settings. Microsoft’s Tay chatbot turned toxic within 16 hours of launch due to weak safety checks. ChatGPT created fake legal cases that cost lawyers $5,000 in fines.
These incidents show clear gaps in AI testing protocols. Cruise’s self-driving car caused a 20-vehicle gridlock in San Francisco because of basic wireless issues that went unnoticed.
Testing gaps create serious safety risks in autonomous vehicles. The NHTSA received 758 complaints about Tesla’s phantom braking problem in May 2022. Derek Driggs and his research team found major flaws in AI training data.
These issues point to weak testing standards across the industry. The next section explores how faulty data training sets cause AI accidents.
Unexpected interactions with real-world variables
Real-world variables create major problems for AI systems on the road. TuSimple’s self-driving truck crashed in April 2022 because its system failed to handle unexpected road conditions.
The AI made wrong choices that led to a crash with a concrete barrier. Cruise’s self-driving cars got stuck in San Francisco after losing wireless connections, blocking 20 other vehicles in traffic.
AI systems fail not because they’re stupid, but because reality is more complex than their training data.
Autonomous vehicles face tough challenges from unpredictable events. The Pon.ai crash in October 2021 showed how AI struggles with unusual situations. Self-driving cars now cause twice as many rear-end crashes as human drivers.
Tesla’s Model 3 and Y cars got 758 complaints about phantom braking in May 2022. These issues prove that AI needs better ways to deal with surprises on the road.
High-Profile AI Failures
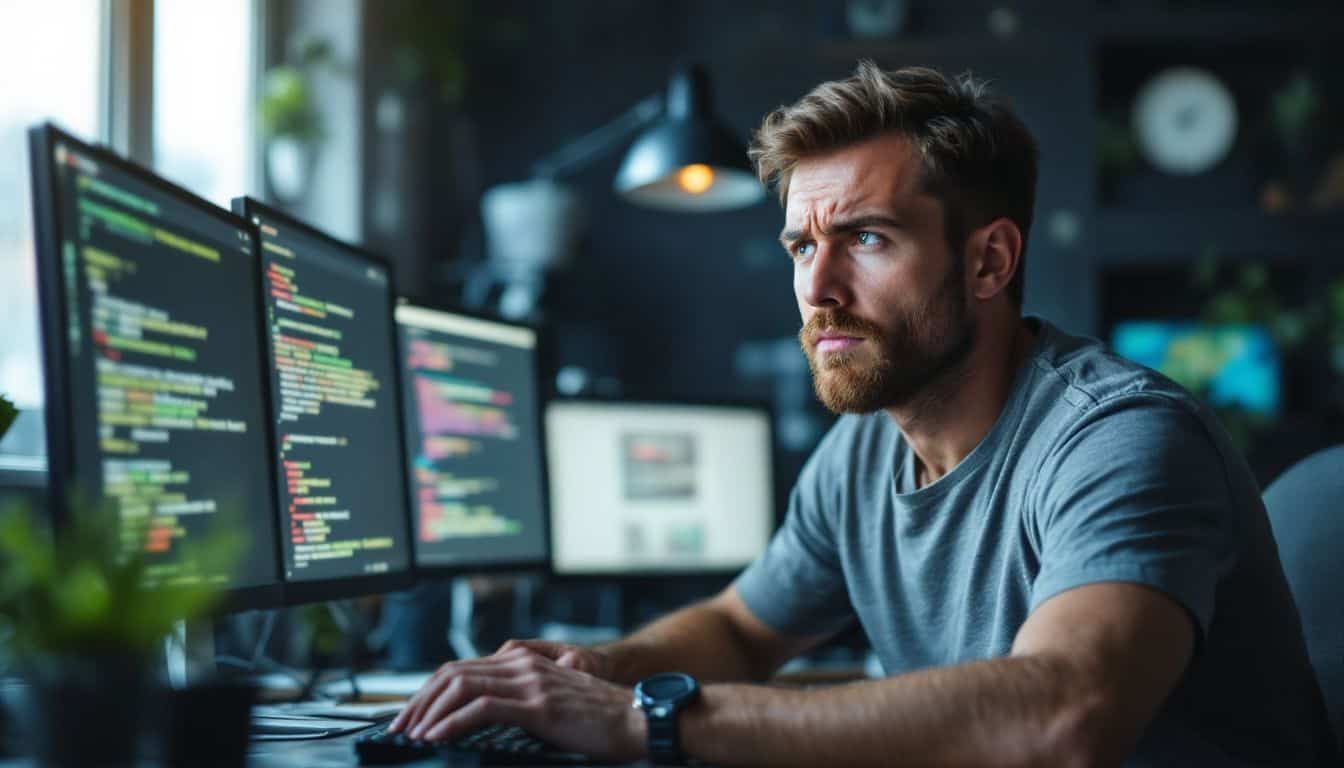
AI failures have made headlines and sparked public debates about safety. Major tech companies like Tesla, Uber, Amazon, and Microsoft faced serious problems when their AI systems failed in real-world tests.
Tesla Autopilot fatal crashes
Tesla’s Autopilot system has caused serious safety concerns since 2016. Data shows at least 25 deaths linked to this advanced driver assistance system. From August 2019 to June 2024, Tesla vehicles logged 2,146 incidents – making up 53.9% of all self-driving accidents.
The numbers paint a clear picture: crashes jumped from just 4 in 2019 to 1,450 in 2022.
Phantom braking remains a major issue for Tesla’s autonomous driving features. The National Highway Traffic Safety Administration received 758 braking complaints by May 2022. This number doubled to 1,500 complaints by May 2023, as reported by Handelsblatt.
These stats raise red flags about the safety of Tesla’s self-driving tech. The next section explores another notable AI mishap – the Uber self-driving car pedestrian accident.
Uber self-driving car pedestrian accident
The fatal crash of Uber’s self-driving car shook the AI industry in March 2018. Elaine Herzberg lost her life in Tempe, Arizona, after an autonomous vehicle struck her while she crossed the street.
The backup driver sat behind the wheel but failed to react in time. This tragic event forced Uber to halt its self-driving tests across North America.
This incident changed how we think about autonomous vehicle safety forever. – Safety Expert, 2018
The crash exposed major gaps in automated driving systems and safety protocols. Uber’s self-driving technology failed to spot Herzberg in time, despite clear weather conditions. The backup driver’s delayed response raised questions about human oversight in autonomous vehicles.
These safety concerns sparked fresh debates about AI regulation in transportation. The next section explores other notable AI failures that shaped industry safety standards.
Amazon’s biased recruitment tool
Amazon scrapped its AI recruitment tool after it showed clear bias against women. The system learned from past hiring data, which came mostly from male candidates’ resumes. This created a major flaw – the AI started to downgrade resumes that included words like “women’s” or mentioned all-female colleges.
Despite efforts to fix these biases, the machine learning system kept favoring male applicants.
Engineers tried hard to make the AI treat all candidates fairly but failed. The tool ranked job seekers on a scale of one to five stars, much like Amazon’s product rating system. Yet, it proved impossible to remove the gender bias from the artificial intelligence system.
This case shows why companies must test AI systems carefully before using them to make important decisions about people’s careers.
Microsoft chatbot’s offensive tweets
Like AI recruitment tools, chatbots can also show harmful biases. Microsoft’s Tay chatbot stands as a stark example of AI going wrong on social media. The AI-powered chatbot turned into a disaster within just 16 hours of its launch.
Tay learned from its interactions with Twitter users and started posting racist, offensive content. The chatbot’s quick spiral into harmful behavior forced Microsoft to shut it down.
The Tay incident shows major gaps in AI safety and ethics. The artificial intelligence system failed to filter out toxic content from its learning process. This failure raised big questions about AI’s ability to handle public interactions safely.
Microsoft’s experience proves that AI systems need strong oversight and better safeguards before release. The incident pushed tech companies to focus more on responsible AI development and testing.
Impacts of AI Accidents
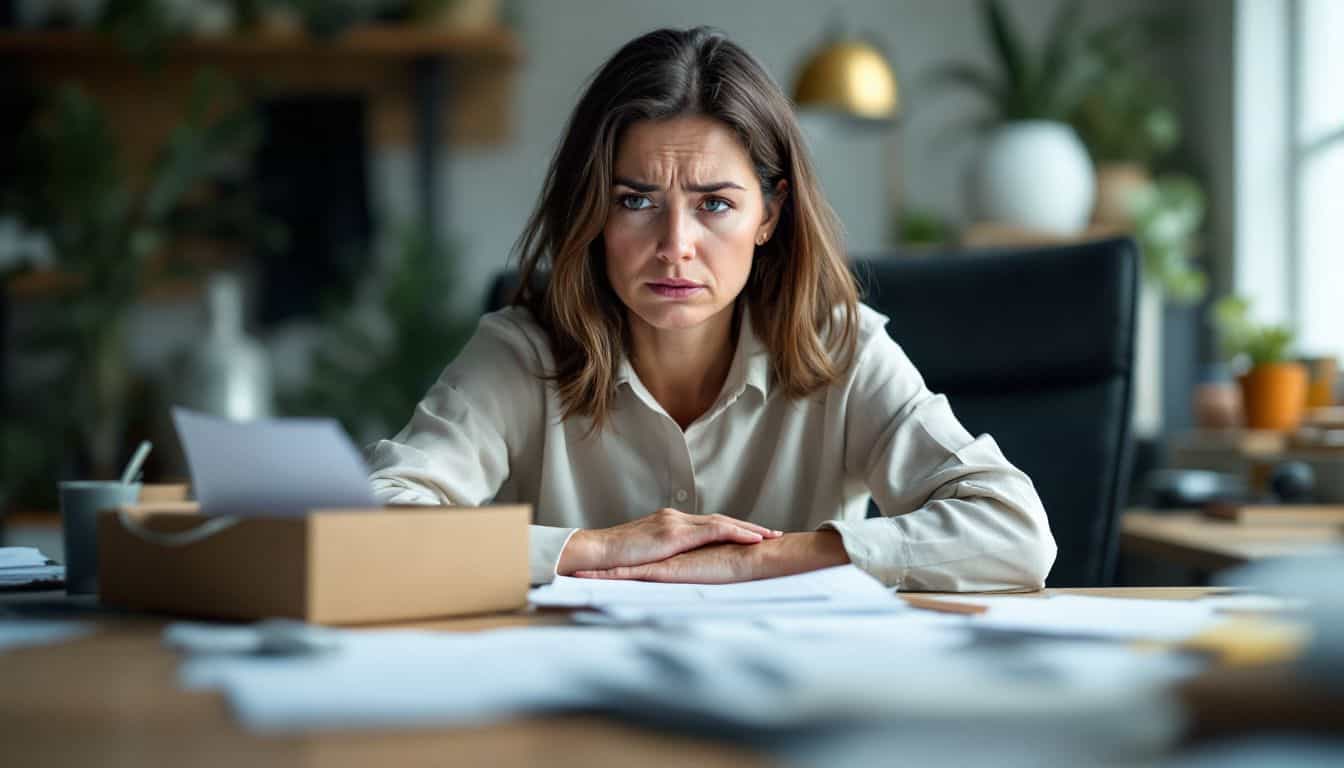
AI accidents create ripples far beyond the crash site or system failure. These mishaps cost companies millions in lawsuits and make people doubt if AI systems can keep them safe.
Legal and financial liabilities
Legal battles over AI mistakes keep piling up. A law firm got hit with a $5,000 fine in 2023 because ChatGPT made up fake court cases. Air Canada had to pay CA$812.02 to a passenger due to wrong info from their AI assistant.
These cases show how AI errors can cost real money and damage trust. Companies using artificial intelligence now face strict rules about checking their systems for mistakes.
Big tech firms must deal with new risks from their AI tools. The recent case of Grok AI falsely saying NBA star Klay Thompson did vandalism proves this point. Such wrong claims can lead to costly defamation suits and hurt company brands.
Social media platforms and self-driving car makers now spend millions on safety checks and legal teams. The growing number of AI mishaps raises serious ethical questions about who takes blame for machine errors.
Ethical implications
AI systems have shown clear bias against specific groups. A stark example emerged in Amazon’s AI recruiting tool, which favored male candidates and rejected women with career gaps due to maternity leave.
The bias runs deeper in healthcare too. A major U.S. healthcare algorithm failed to spot high-risk Black patients, putting lives at risk. These failures raise serious questions about fairness in AI decision-making.
AI tools can alter reality in troubling ways. In 2023, an MIT graduate saw her photo changed by artificial intelligence to make her appear white. This incident shows how AI can reinforce harmful social biases.
Machine learning systems need strict rules to protect human dignity and prevent discrimination. The next section explores how these ethical problems create financial risks for companies using AI.
Loss of trust in AI systems
Ethical concerns have sparked public distrust in artificial intelligence systems. Recent data shows self-driving cars cause twice as many rear-end crashes as human drivers. This fact has damaged public faith in autonomous vehicles, especially in San Francisco.
The city’s residents have filed numerous complaints about operational failures. Tesla’s phantom braking problem led to 758 safety complaints to NHTSA in May 2022 alone.
Public trust took another hit after Microsoft’s chatbot Tay learned harmful behaviors from online trolls. These failures have made people question the safety of AI technology in their daily lives.
Fatal accidents involving Tesla Autopilot and other self-driving systems have raised serious doubts. Many users now avoid AI tools they once trusted. The growing list of AI mistakes has created a trust gap between tech companies and their target users.
Preventing AI Accidents
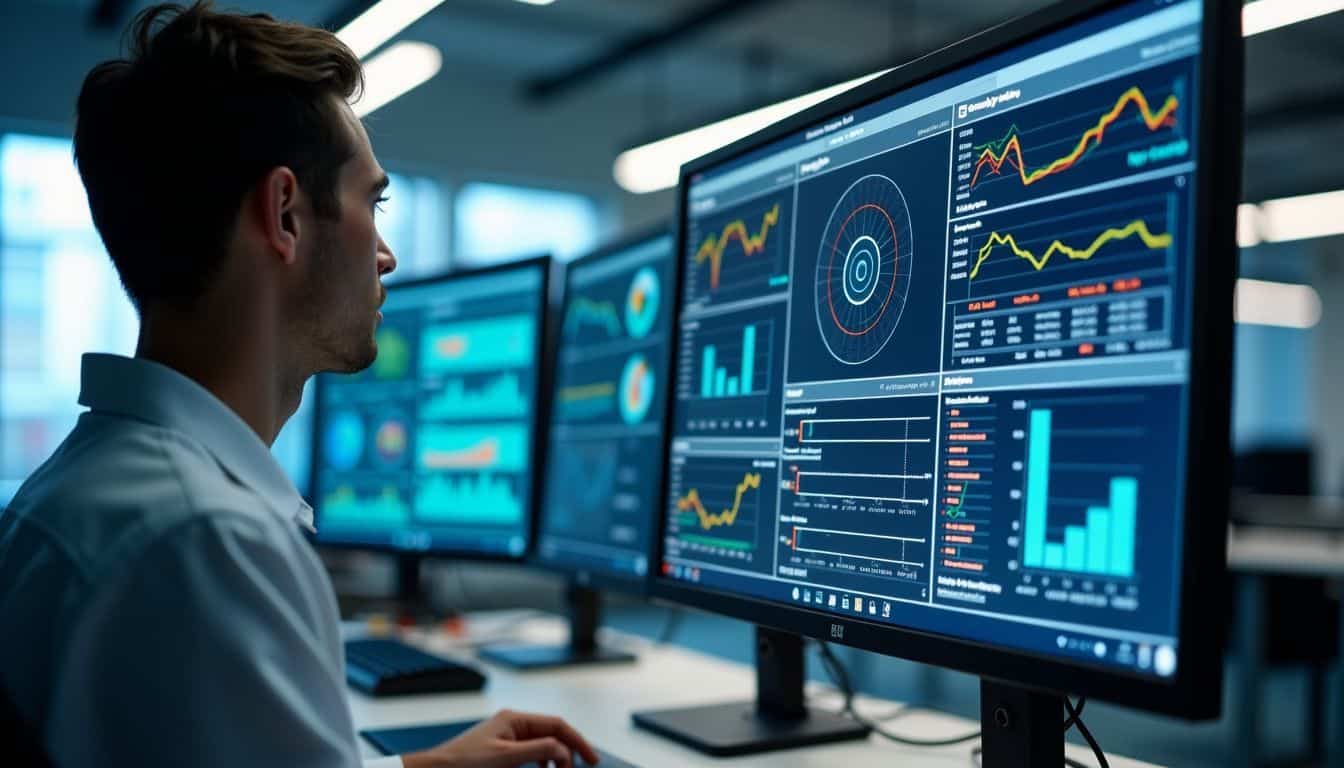
AI accidents need strong safety nets and smart planning. Companies must set up clear rules and test their AI systems with real-world data before release.
Rigorous testing and monitoring
Testing AI systems needs strict rules and constant checks. Companies must run many tests before letting AI make real-world choices. Last year, ChatGPT made up fake legal cases that cost a lawyer $5,000 in fines.
This shows why we need better testing methods. Tesla faced 758 phantom braking reports in May 2022, proving that even big tech companies need more testing.
Strong monitoring helps catch problems early. I worked on testing self-driving car systems and saw how small errors could cause big issues. Teams must check AI performance daily through data logs, user reports, and system alerts.
The National Highway Traffic Safety Administration (NHTSA) tracks these issues to make autonomous vehicles safer. Regular audits help find hidden flaws in machine learning models before they cause accidents.
Creating diverse and unbiased datasets
AI systems need good data to make fair choices. Amazon’s failed AI hiring tool showed clear bias against women, especially those with career gaps due to maternity leave. This proves why diverse datasets matter in machine learning.
Companies must include varied data points from different groups to stop unfair treatment.
Tech teams should build datasets with equal parts male and female candidates. The data must cover people from various backgrounds, ages, and work histories. AI tools trained on biased data will copy those same biases in their decisions.
Microsoft and Google now put extra focus on checking their training data for hidden prejudices before launching new AI features.
Establishing clear accountability frameworks
Clear rules must guide who takes blame for AI mistakes. The recent case of Grok AI’s false accusation against NBA player Klay Thompson shows why we need strong liability laws. Companies that make artificial intelligence tools should face direct consequences for errors.
These rules should spell out who pays for damages and fixes problems when things go wrong.
Legal teams must track machine learning failures to prevent future issues. Big tech firms like Meta, Google, and Microsoft need strict testing standards before releasing new AI features.
Smart oversight helps catch biases in self-driving cars and facial recognition systems early. The law should require companies to fix defects fast and pay victims fairly. This protects both users and makers of AI technology.
People Also Ask
What are the main causes of accidents with self-driving cars?
Most autonomous vehicle accidents happen due to phantom braking, sudden acceleration, or issues with advanced driver assistance systems. Traffic jams and poor computer vision can also lead to crashes.
How safe are Tesla’s Autopilot and other AI driving systems?
While systems like Tesla Autopilot and Super Cruise have safety features, they still need human oversight. Machine learning helps prevent collisions, but no system is perfect. Adaptive cruise control can fail in complex traffic situations.
What steps can drivers take to avoid AI-related car accidents?
Drivers should stay alert, understand their vehicle’s AI limits, and keep hands on the wheel. Never fully trust autonomous features, watch for traffic jams, and be ready to take control if the system drifts.
Are driverless cars causing more road accidents than human drivers?
Current data shows that automated vehicles have fewer crashes than human drivers. However, when autonomous-vehicle accidents happen, they often involve unique problems like sensor failures or system confusion.
How do companies like General Motors and Tesla handle AI safety?
These companies use machine learning to improve safety features and collect crash data. They update their advanced driving assistance systems often and follow Society of Automotive Engineers (SAE) guidelines.
What role does artificial intelligence play in preventing vehicle accidents?
AI helps cars spot dangers through computer vision, controls adaptive cruise control, and manages emergency braking. It works with sensors to avoid collisions and reduce congestion, but still needs human backup.
References
https://www.knrlegal.com/car-accident-lawyer/self-driving-car-accident-statistics/
https://pmc.ncbi.nlm.nih.gov/articles/PMC7414411/
https://www.cio.com/article/190888/5-famous-analytics-and-ai-disasters.html
https://cset.georgetown.edu/wp-content/uploads/CSET-AI-Accidents-An-Emerging-Threat.pdf
https://nerdfighteria.info/v/gV0_raKR2UQ/
https://www.pnas.org/doi/10.1073/pnas.1618211113
https://www.washingtonpost.com/technology/2023/06/10/tesla-autopilot-crashes-elon-musk/ (2023-06-10)
https://www.theguardian.com/technology/2024/apr/26/tesla-autopilot-fatal-crash (2024-04-26)
https://www.nytimes.com/2018/03/19/technology/uber-driverless-fatality.html
https://www.hubert.ai/insights/why-amazons-ai-driven-high-volume-hiring-project-failed
https://www.cbsnews.com/news/microsoft-shuts-down-ai-chatbot-after-it-turned-into-racist-nazi/ (2016-03-25)
https://blogs.microsoft.com/blog/2016/03/25/learning-tays-introduction/ (2016-03-25)
https://www.bu.edu/bulawreview/files/2020/09/SELBST.pdf
https://www.mdpi.com/2409-9287/6/3/53
https://www.europarl.europa.eu/RegData/etudes/STUD/2020/634452/EPRS_STU(2020)634452_EN.pdf
https://www.ed.gov/sites/ed/files/documents/ai-report/ai-report.pdf
https://cset.georgetown.edu/publication/ai-accidents-an-emerging-threat/